
Title
MWPCR: Multiscale Weighted Principal Component Regression
Introduction
Multiscale weighted principal component regression (MWPCR) is a framework for the use of high dimensional features with strong spatial features (e.g., smoothness and correlation) to predict an outcome variable, such as disease status. This development is motivated by identifying imaging biomarkers that could potentially aid detection, diagnosis, assessment of prognosis, prediction of response to treatment, and monitoring of disease status, among many others.Questions
1. Can we correctly integrate the spatial information in the principal component analysis?2. Can we reduce the dimension of the big data and detect important regions among millions of voxels?
Methods
The MWPCR can be regarded as a novel integration of principal components analysis (PCA), kernel methods, and regression models. In MWPCR, we introduce various weight matrices to prewhitten high dimensional feature vectors, perform matrix decomposition for both dimension reduction and feature extraction, and build a prediction model by using the extracted features. Examples of such weight matrices include an importance score weight matrix for the selection of individual features at each location and a spatial weight matrix for the incorporation of the spatial pattern of feature vectors. We integrate the importance score weights with the spatial weights in order to recover the low dimensional structure of high dimensional features. Our package can be found at https://www.nitrc.org/projects/mwpcr/.Findings
Our models can clearly identify the key important regions in the noisy images. When applied in both classification and regression problems, our method outperforms other "off-the-shelf" high dimensional classification or regression methods. The following is the 4 leading PC loadings of the ADNI PET data in the AD v.s. NC classification problem.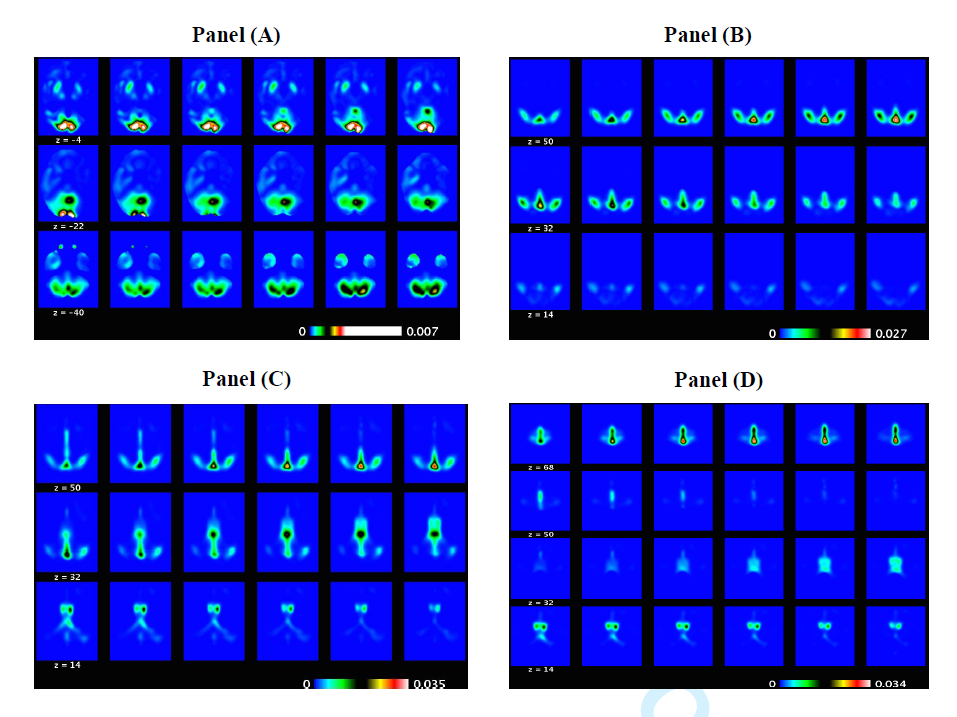
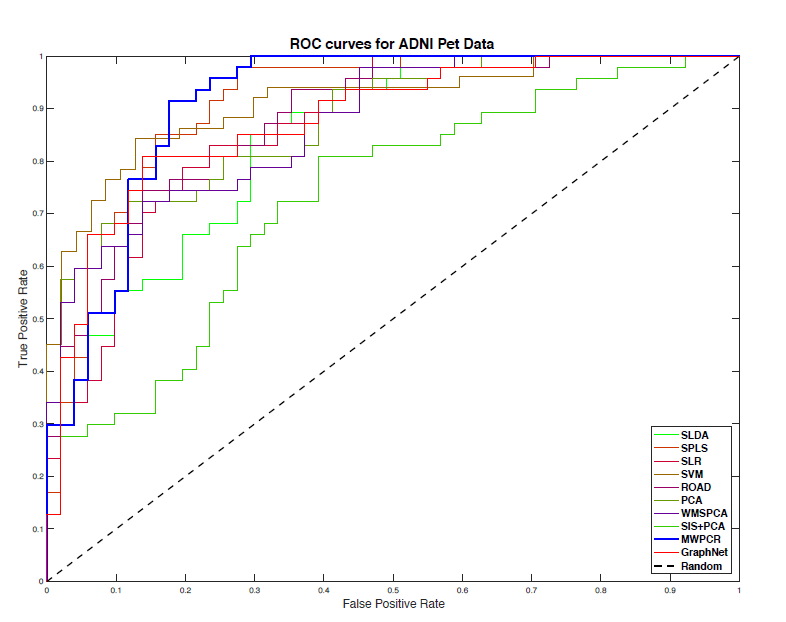
References
1. Zhu, HT., Shen, D., Peng, X. W. and Leo Liu YF. Multiscale weighted principal component analysis for high-dimensional data on graphs. Journal of American Statistical Association, in press, 2016.2. Li, Yimei, et al. "Multiscale adaptive regression models for neuroimaging data." Journal of the Royal Statistical Society: Series B (Statistical Methodology) 73.4 (2011): 559-578.