
Title
Discovering Genetic Markers Associated with Human Brain Development using Diffusion Tensor Image Data
Introduction
In a typical diffusion tensor Imaging (DTI) study, diffusion properties are observed among multiple fiber bundles to understand the association between neurodevelopment and clinical variables, such as age, gender, biomarkers, etc. Most research focuses on individual tracts or use summary statistics to jointly study a group of tracts, which usually ignores the global and individual functional structures. To address this problem, we propose a hierarchical functional principal regression model to jointly analyze functional data and to extract effect on both global and individual levels.Methods
The hierarchical functional principal regression model (HPRM) consists of three major components: (i) a multidimensional Gaussian process model to characterize functional data, (ii) a latent factor model to jointly analyze multiple fiber bundles to capture both common feature and individual feature, and (iii) a multivariate regression model to study global effect as well as individual effect. A multilevel estimation procedure is proposed and a global statistic is introduced to test hypothesis of interest.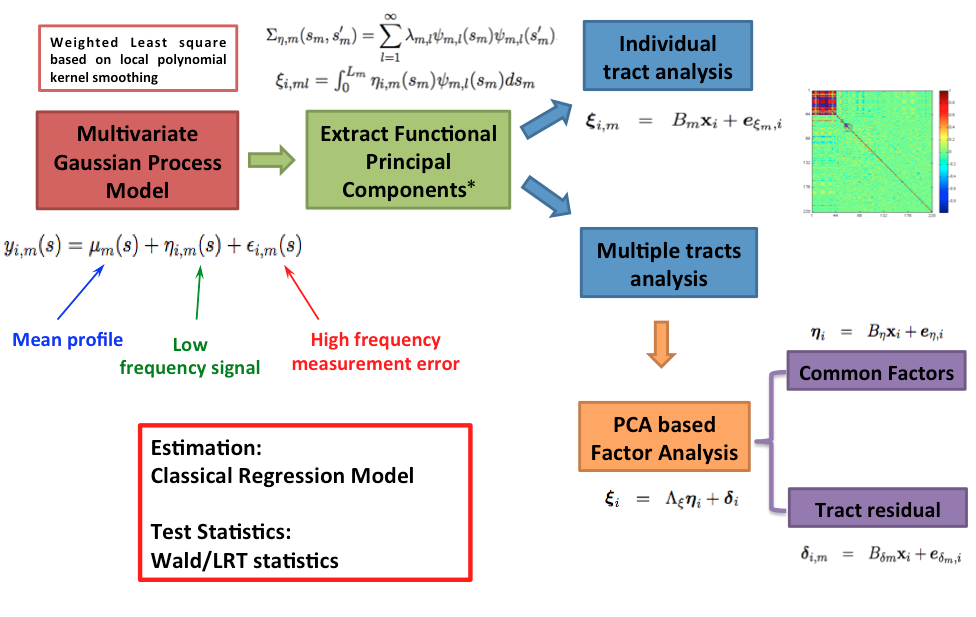
Findings
To explore genetic markers contributing to white matter microstructure development during prenatal period, HPRM is applied to a neonate DTI study with FA measure of 44 fiber tracts. Functional PCA is applied to each individual tract and five PC scores are extract respectively to include more than 75% of total variation. In factor analysis, the first factor explains more than 49% of total variation and each of the rest factors explains less than 5%. Therefore, the first factor is extracted as common factor. GWAS result on common factor shows that snps on ALK gene are strongly associated with the common factor. Single tract analysis was conducted as a comparison. For the top markers in ALK gene, pvalues are small for a large proportion of fiber tracts, but not significant enough in GWAS. This suggests HPRM is much more powerful to detect weak genetic signal when there is shared effect. Also, we are able to identified some other important genetic markers known to be associated with brain development and brain abnormality.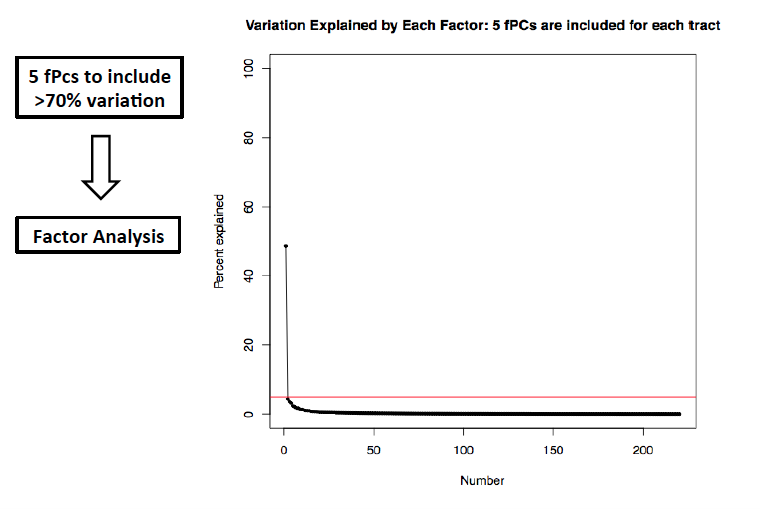
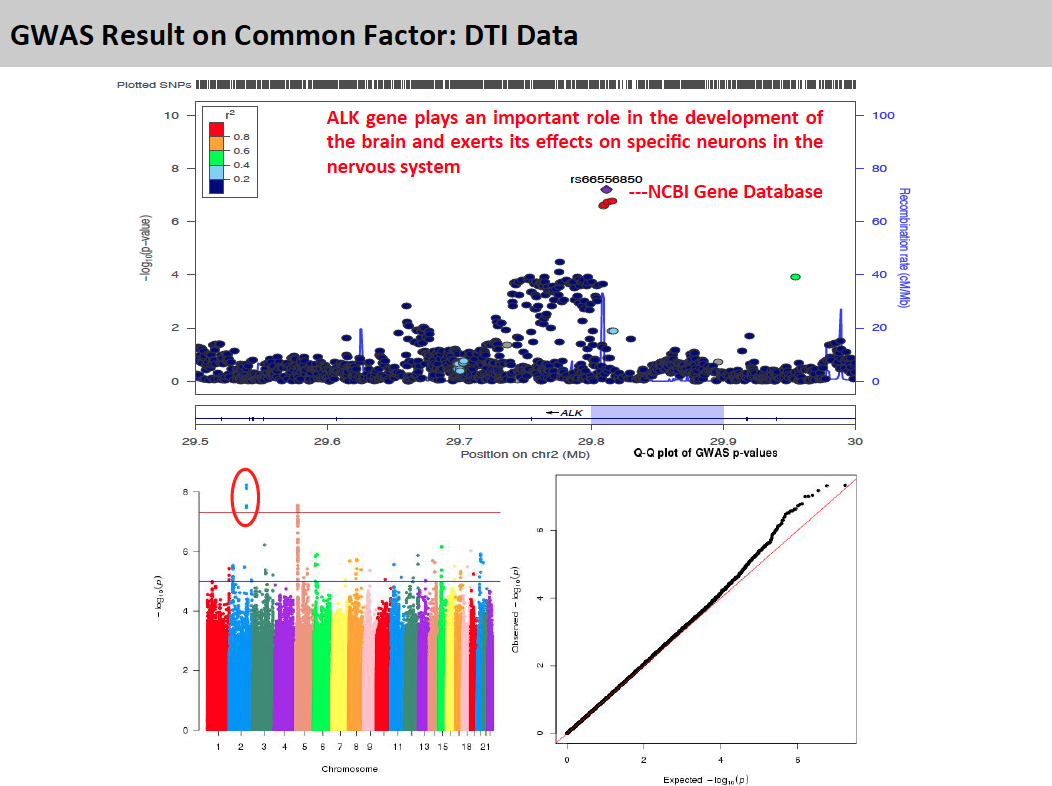
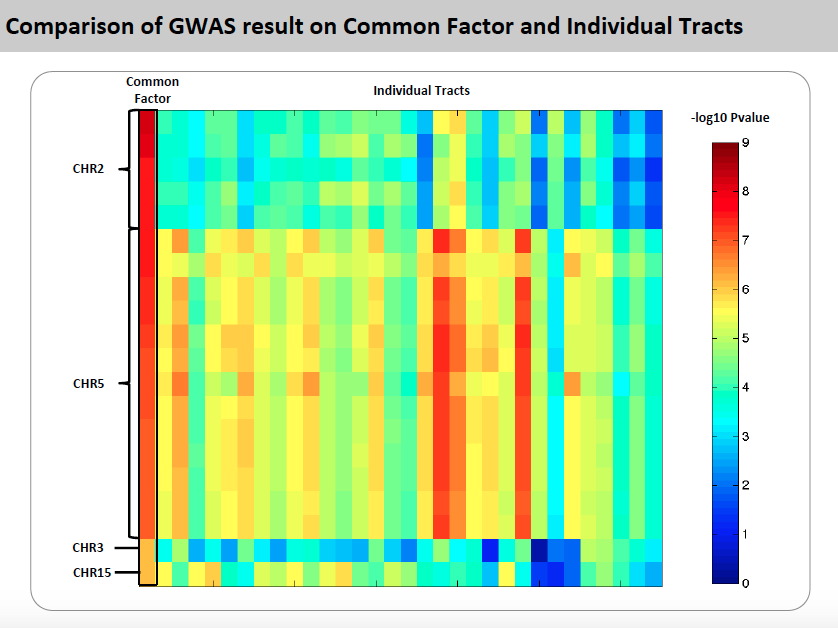
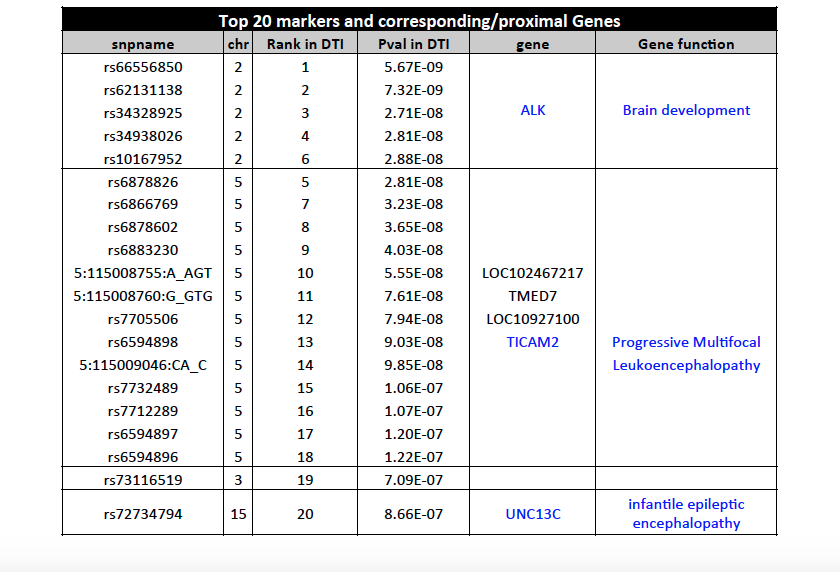
References
[1] Zhu, Hongtu, Linglong Kong, Runze Li, Martin Styner, Guido Gerig, Weili Lin, and John H. Gilmore. "FADTTS: functional analysis of diffusion tensor tract statistics." NeuroImage 56, no. 3 (2011): 1412-1425.[2] Zhang, Jingwen, Huang Chao, Rebecca C. Knickmeyer, John H. Gilmore, Joseph Ibrahim and Hongtu Zhu. "Hierarchical Principal Regression Model for Diffusion Tensor Image Statistics." (Manuscript)