
Title
Human papilloma virus (HPV) prediction from CT images
Introduction
Human papilloma virus (HPV) associated cancers have been shown to have increased survival and better tumor control with radiotherapy than non-HPV-associated cancers. HPV status is predictive of outcomes, and is tested routinely using immunohistochemistry for p16, a protein, or in situ hybridization for viral DNA. Recent data suggest that "radiomics", or extraction of image texture analysis to generate mineable quantitative data from medical images, can reflect phenotypes for various cancers. Several groups have shown that developed radiomics signatures, in head and neck cancers among other tumor sites, can be correlated with survival outcomes. The University of Texas MD Anderson Cancer Center (MDACC) provided dataset of anonymized DICOM files represent a realtively uniform cohort of 315 oropharynx cancer patients, supplemented with relevant clinical data, known etiological/biological correlates (specifically, human papilloma virus "HPV" status) as ground truth. Our major target is to assess the ability of participant-developed radiomic workflows to predict binary (phenotypic/genotypic) HPV status, using a defined “Training” cohort as a "prior" dataset that includes all input and outcome data, to build up an algorithm.Questions
Using expert-segmented contrast-enhanced computed tomography (CT) images to predict whether a tumor is HPV positive (as defined by p16 or HPV testing).Methods
First, we extracted the global radiomics features from each ROI segmented by radiologists and checked the quality of features between training and testing subjects to ensure the similarity of the distributions of the features between training and testing cohort. Then, we picked the features that were differentially distributed between recurrence subjects of the training cohort and non-recurrence ones. The correlation between these differentially distributed features and the responses (which is recurrence and non-recurrence) were then calculated to narrow down the candidate features. Last the features were ranked by the prediction performance when built model with the feature alone. the final model was then picked by a forward selection method.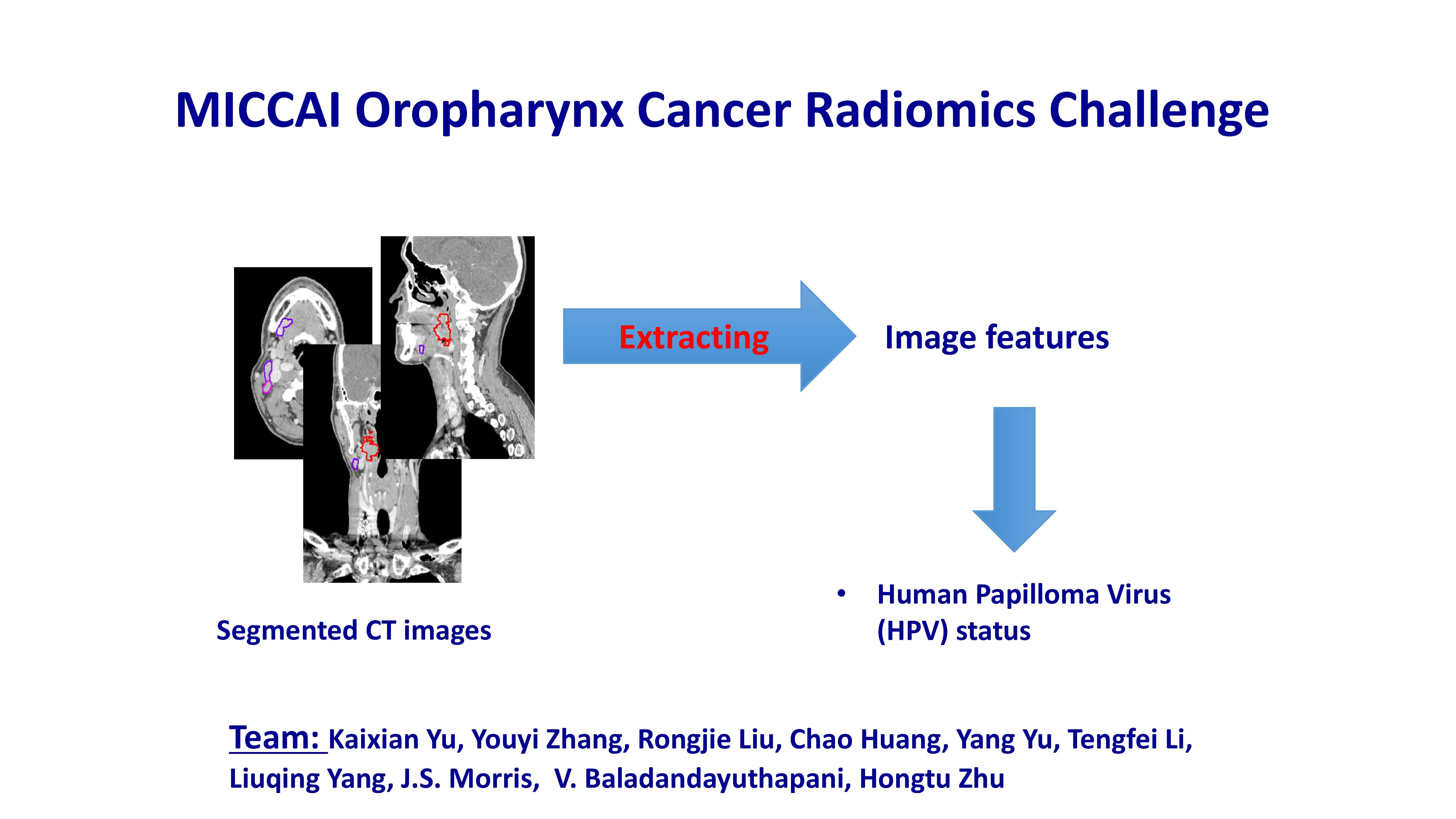
Findings
Two features (Mean Breadth and Spherical Disproportion) were finally selected. Mean breadth is the mean “width” of the ROI, while spherical disproportion is the ratio of the surface area of the image ROI to the surface area of a sphere with the same volume as the image ROI. We found that, for HPV+ subjects, both mean breadth and spherical disproportion tend to have smaller values. For the testing cohort, based on our method, the area under the receiver operator characteristic curve (ROC AUC) of HPV status achieved 0.915, which means our proposed workflow can detect some potential radioimcs biomarkers for HPV associated cancer prediction. In addtion, we have finished in the first place in the MICCAI Radiomics Challenge (MICCAI).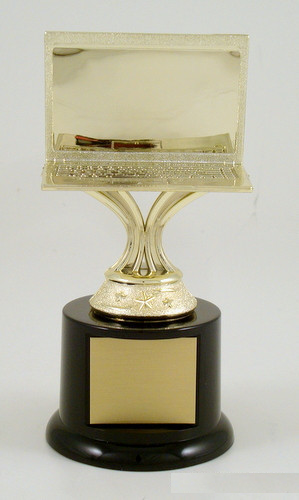