
Title
Population-Based Structural Connectome Analysis
Introduction
Many challenging issues, such as statistical variability in a population, arise from the study of structural connectome maps by using diffusion MRI tractography data. Addressing these challenges requires the development of fast and reliable approaches for processing high-dimensional diffusion data from hundreds (or even thousands) of subjects. We aim to develop a reliable Population-based Structural Connectome (PSC) Mapping framework to construct population structural connectome maps on a common space (or template) while accounting for individual variabilities. Our goal is to provide a set of tools for researchers to conveniently explore structural connectivity. These tools will allow one to construct and visualize individual structural connectome in different forms, from coarse to detail, e.g. (1) binary network, (2) weighted network, (3) tensor network and (4) streamline-based connnectome. At the binary network level, the connection two regions of interest are represented as 0 (not connected) or 1 (connected). At the weighted network level, a scalar number is assigned to indicate the "connection strength" of these two regions. At the tensor network level, a set of scalar numbers is assigned to characterize this connection. Eventually, at the streamline level, the connection is characterized by the reconstructed fibers, which gives the most detailed information about this connection.Questions
There are two key questions we try to answer: (1) How to reliably construct the connectome for population-based analysis? (2) How to efficiently summarize the data to allow meaningful statistical analysis for a population of individuals?Methods
The whole pipeline includes five different modules as illustrated in Figure 1. Module (1) and (2) use state-of-the-art tractography algorithm to reconstruct the fiber curves using dMRI and structural MRI. Module (3), (4) and (5) are newly developed procedures for reliably extract each connection and efficiently represent each connection. The outputs of this pipeline are: binary networks, weigthed networks, tensor networks and streamline connectivity matrices.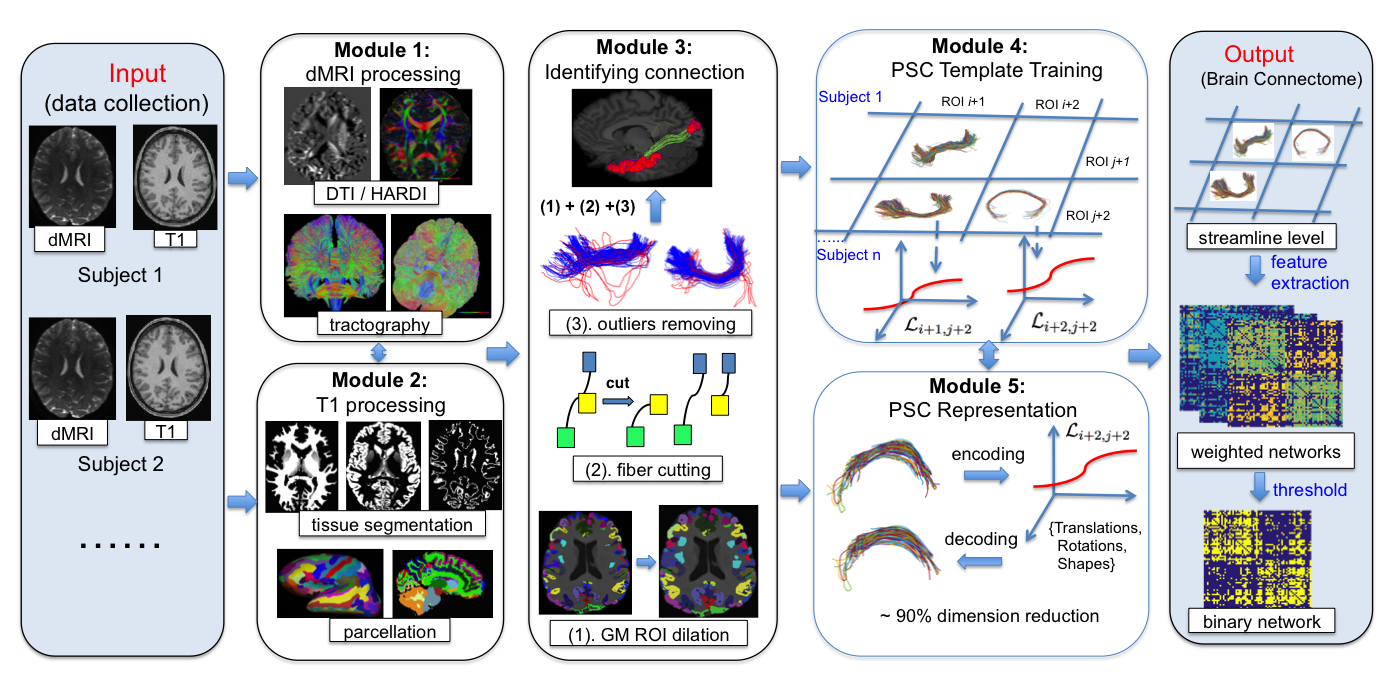
Findings
The developed PSC allows one to perform population based structural connectivity analysis. Figure 2 shows the binary and weighted network extracted from PSC.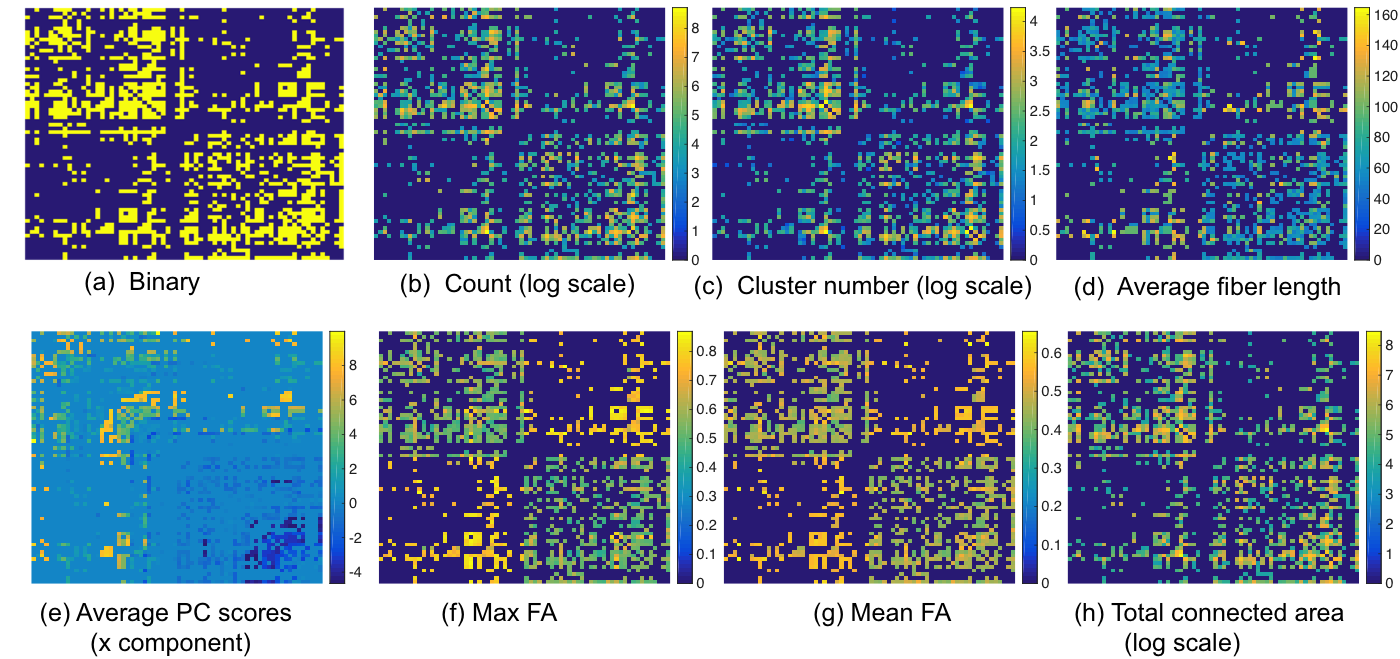
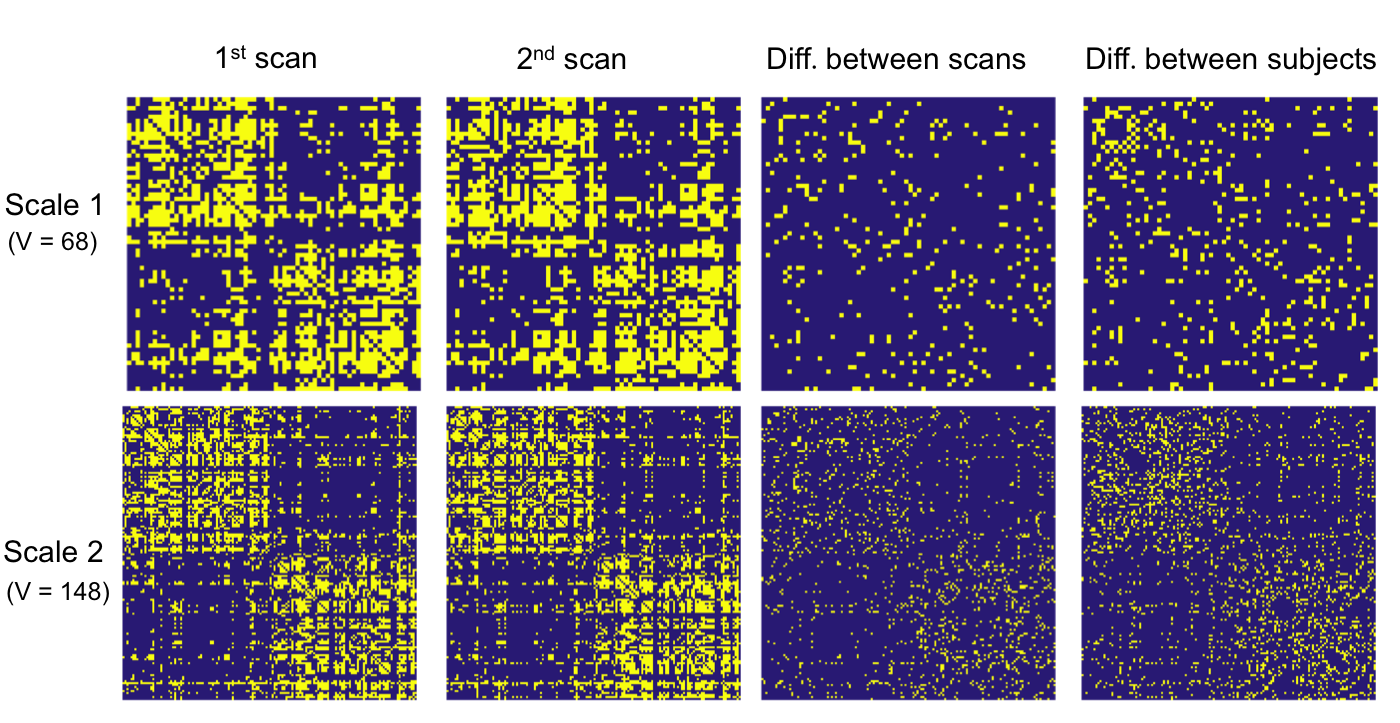
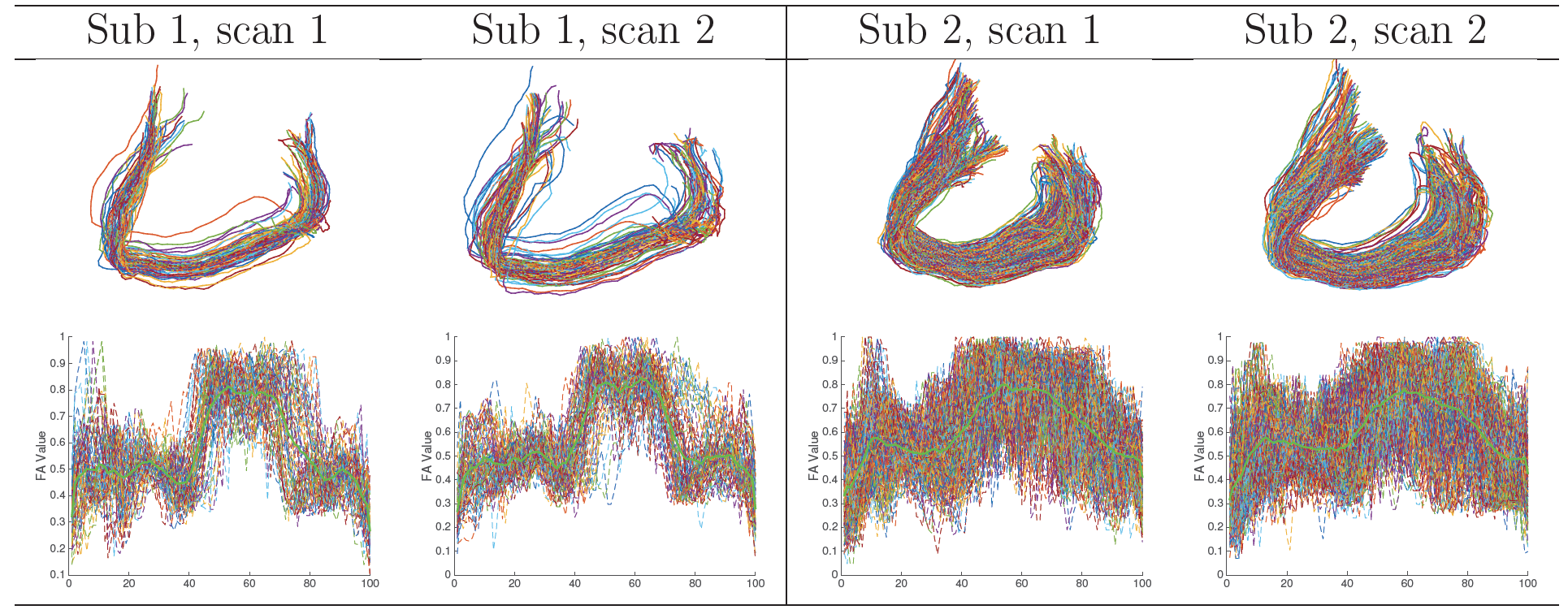
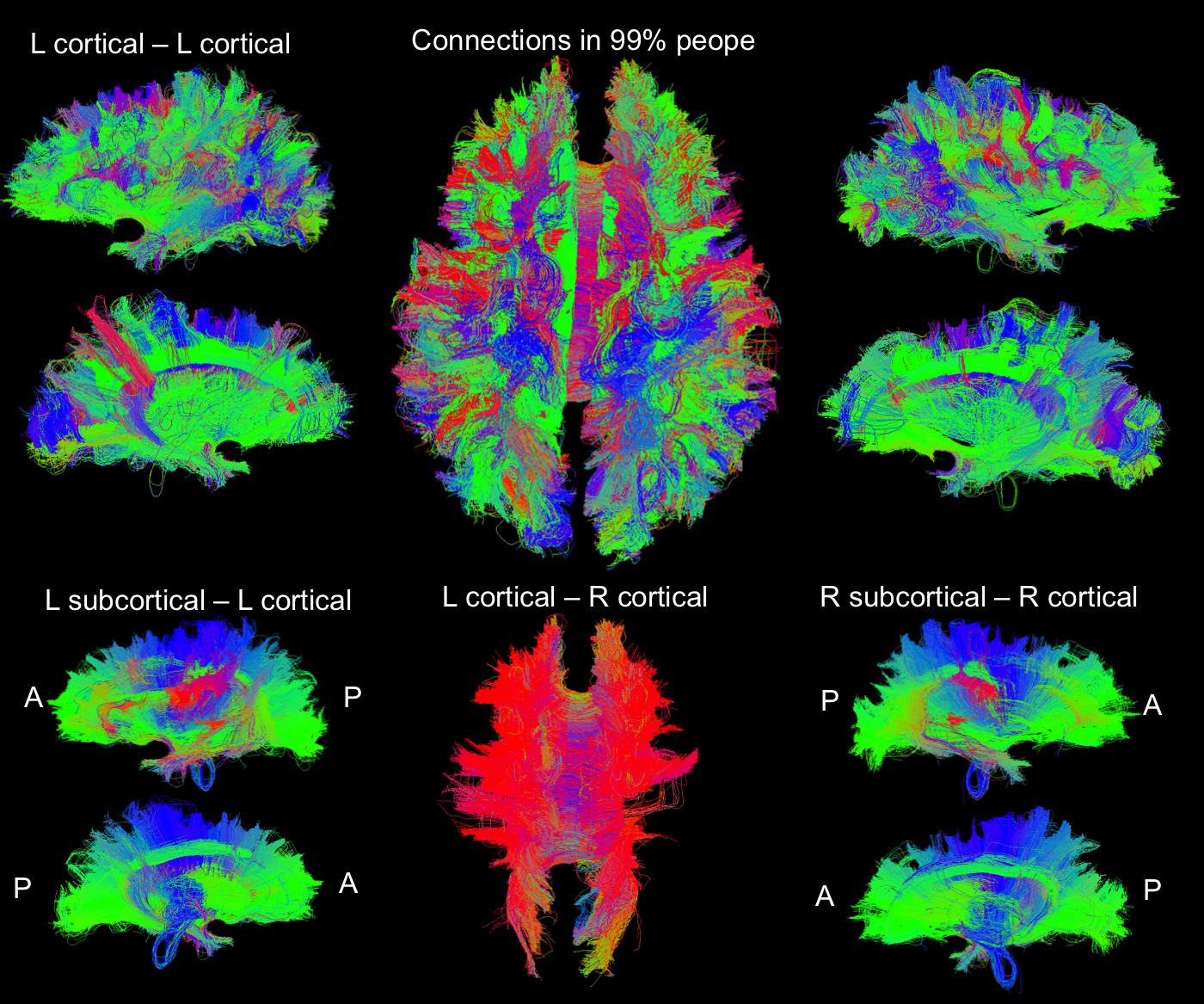