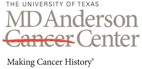
Han Liang's Research Group
AI, Big data, Cancer, Drug development
-
The fundamental question driving our research paradigm is how to take full advantage of cancer genomic data to elucidate the molecular basis of human cancer and develop effective therapeutic strategies, thereby contributing to the true promise of personalized or precision cancer therapy. Combining both computational and experimental approaches, my group research focuses on the following areas.
-
AI-driven bioinformatic tool development
One key goal of our group is to allow a broad research community to easily generate testable hypotheses and obtain biological insights from high-throughput genomic data. Over the past several years, we have developed several popular bioinformatics tools, including. (i) TCPA: an integrative bioinformatic data portal for visualizing and analyzing cancer reverse-phase protein array-based functional proteomic data (Li et al. Nat Methods; Li et al. Cancer Res 2017). (ii) TANRIC: an interactive open platform to explore the function of lncRNAs in cancer (Li et al. Cancer Res 2015). (iii) DrBioRight: the first nature language-oriented, AI-driven analytics platform for omics data (Li et al. Cancer Cell 2020). These tools collectively have >110,000 users from >100 countries. -
Comparative genomics analysis across cancer types
Our group is one major contributor to large cancer genomics projects such as TCGA and ICGC. We systematically assessed the prognostic and predictive utility of TCGA genomic and proteomic data (Yuan et al. Nat Biotechnol 2014). We performed the first global analysis of sex effects on the molecular profiles of cancer patients and identified two classes of sex-effect cancer types (Yuan et al. Cancer Cell 2016). We performed a systematic analysis of hypoxia-related molecular features and their clinical values in terms of selecting cancer therapies (Ye et al., Nat Metab 2019). Collaborating with an international team, using ICGC whole-genome sequencing data, we performed a comprehensive molecular characterization of mitochondrial genomes in human cancers (Yuan et al. Nat Genet 2020). -
Function and clinical utility of enhancer RNAs in cancer
Using TCGA RNA-seq data, we performed the first pan-cancer analysis of expressed enhancer RNAs and elucidated the enhancer-mediated regulatory mechanism of PD-L1 (Chen et al. Cell 2018). We demonstrated that fast-evolving human-specific enhancers are associated with cancer genes due to antagonistic pleiotropy (Chen et al. Cell Syst 2018). We constructed a high-resolution map of human enhancer RNA loci, enabling the quantification of super-enhancer activities (Chen ant Liang, Cancer Cell 2020). These results highlight regulatory RNAs expression analysis as a new paradigm for investigating cancer mechanisms and discovering prognostic biomarkers. -
Function and clinical utility of RNA modifications in cancer
RNA editing is an important post-transcriptional mechanism that can cause “mutations” at the RNA level. We have systematically characterized the A-to-I RNA editing events and demonstrated their clinical relevance in human cancer (Han et al. Cancer Cell 2015) as well as RNA editing hotspots in miRNAs (Wang et al. Genome Res 2017). We demonstrated a significant contribution of RNA editing to proteomic diversity (Peng et al. Cancer Cell 2018). We characterized the clinical patterns and molecular mechanisms of edited miR-379 and highlighted its potential as a pan-cancer small RNA therapeutic (Xu et al. J Clin Invest 2019). -
Functional genomics/proteomics approaches for precision cancer medicine
With our collaborators, we developed a systems-biology approach to identifying cancer driver mutations by combining exome sequencing, high-throughput cell viability assays, and high-throughput protein expression arrays, which addresses a long-standing challenge in the field of cancer genomics (Li et al. Cancer Cell 2017; Ng et al. Cancer Cell 2018). We assessed the performance of different computational algorithms in predicting cancer driver mutations (Chen et al. Genome Biol 2020). Based on perturbed functional proteomics data, We developed a systems-biology approach to identify combination therapies based on "adaptive responses" (Zhao et al. Cancer Cell 2020).