Statistical Bioinformatics Lab
Wenyi Wang received her PhD in Biostatistics (Johns Hopkins University, 2007) and a joint postdoctoral training at Stanford Genome Technology Center and UC Berkeley Statistics (2007-2010). In 2010, she joined the Department of Bioinformatics and Computational Biology at the University of Texas MD Anderson Cancer Center. Wenyi's research includes contributions to statistical bioinformatics in cancer, including MuSE for subclonal mutation calling, DeMixT for transcriptome deconvolution, Famdenovo for de novo mutation identification, and more recently, a pan-cancer characterization of genetic intra-tumor heterogeneity in subclonal selection. Her group is focused on the development and application of computational methods to study the evolution of the human genome as well as the cancer genome, and to further develop risk prediction models to accelerate the translation of biological findings to clinical practice.
Pre-doctoral and post-doctoral fellow positions are available (see the biostatistics position and the cancer genomics position). Please inquire with Dr. Wang.
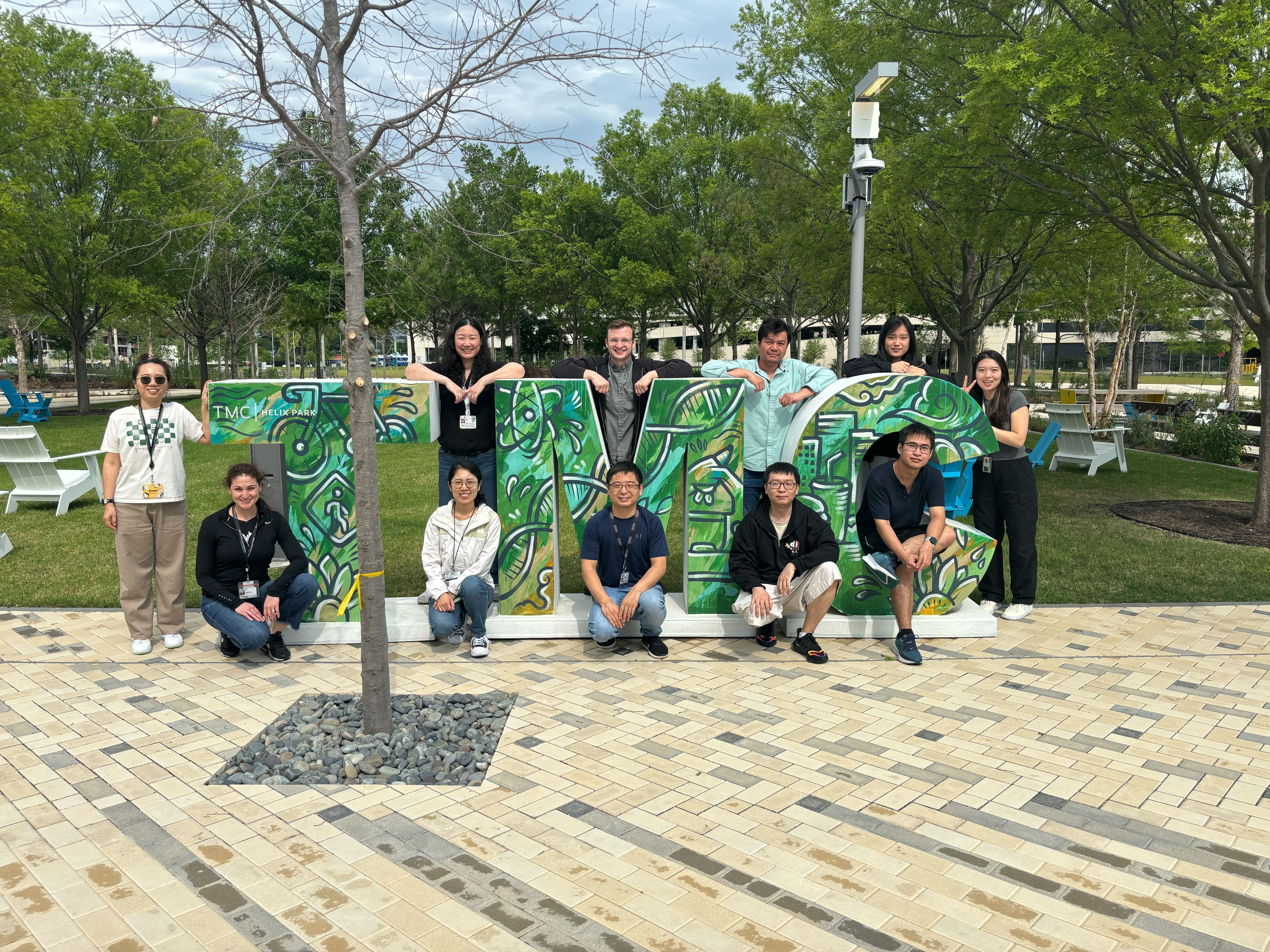
Lab Event May 2024
Current Research Directions
Deconvolution and single-cell modeling for intra- and inter- tumor heterogeneity
Tissues contain many distinct cell types in order to achieve their necessary functions within an organism. The activities of these cell types are determined by their transcriptional patterns and, thus, can be characterized by studying RNA expression data. The same holds true for tumors; as such, there have been many attempts to utilize transcriptomic data to uncover new insights about cancer. Although highly useful for studying and characterizing tumors, single-cell RNA–sequencing (scRNA-seq) data are costly and technically challenging to produce and, thus, often infeasible to use in clinical or research settings at a large scale. Therefore, bulk RNA-seq provides an attractive alternative, as it is significantly more cost-efficient than single-cell methods. However, this bulk data lacks the ability to distinguish between signals from different cell types, which can make downstream analysis difficult or inaccurate. Deconvolution methods have been developed to separate these signals in bulk data, broadening the range of applications in which bulk data can be used. For example, by tracking cell proportions across disease progression, it is possible to use deconvolved bulk data to gain a better understanding of the mechanisms that may cause the disease [DeMixSC]. In cancer research specifically, deconvolution is often used to determine the proportion of a tumor sample that consists of tumor and non-tumor cells or the degree to which different cell types contribute to a tumor’s characteristics. Such analyses have yielded valuable insights into cancer pathways, prognosis, treatment response, and intra- and inter-tumor heterogeneity [DeMixT, TmS] that can be used to improve treatment and outcomes for patients.
Mutation calling and subclonal reconstruction
Cancer is caused by genetic mutations, including single nucleotide variations (SNV), copy number alterations (CNA), and structural variations (SV). These alterations determine the behavior of individual tumors, including their rate of proliferation, resistance to treatment, and potential for metastasis. Therefore, the ability to identify and characterize mutations in tumors is necessary for cancer research and clinical translation. Whole-genome sequencing (WGS) and whole-exome sequencing (WES) data are commonly used in mutation calling efforts, the former because it provides high resolution to detect all mutations and the latter due to its small size and lessened computational requirements. However, many current methods for mutation calling require a significant amount of time to run, making large-scale analysis of genetic alterations difficult. As a result, Dr. Wang’s lab is interested in developing improved mutation calling methods that are computationally efficient [MuSE2]. Such improvements enable the analysis of large volumes of data, which could in turn advance precision medicine and allow novel discoveries to be made about cancer development. Similar advancements are also being made through the reconstruction of the subclonal architectures of tumors – that is, the number and characteristics of subpopulations of cancer cells within individual tumors. Understanding subclonal architecture is critical, as the resulting genetic variation can confer treatment resistance and improved fitness to cancer cell populations. Therefore, Dr. Wang’s lab is investigating new methods to reconstruct subclonal architecture and evolution such that analyses can be conducted more efficiently and with greater accuracy. Specifically, her lab is focusing on addressing the weaknesses of previous methods, such as their lack of intra-tumoral heterogeneity characterization across different cancer types or reliance on extensive computational resources and prior knowledge [Characterizing ITH, CliPP]. The insights gained from mutation calling and subclonal reconstruction analyses could help to improve patient outcomes by providing researchers and clinicians with a deeper understanding of the mechanisms that underlie cancer development and progression.
Semi-parametric survival modeling for cancer risk prediction
Cancer survivors represent a fast-growing yet under-studied population in regard to cancer risk. Second primary cancers, or new cancers that arise in cancer survivors, occur fairly often, particularly for survivors of breast or bladder cancer. However, because risk has not been accurately assessed among cancer survivors, previous cancers are often not considered in cancer prevention strategies. Further, conducting such assessments with pre-existing data is difficult and may be biased, as large pan-cancer databases such as SEER do not account for covariates other than age and sex. To overcome these difficulties, Dr. Wang’s lab examines data from patients affected by Li Fraumeni syndrome (LFS), a heritable condition that increases one’s risk of developing cancer. This population in particular is useful to study because patients can present a wide variety of cancer types and are more likely to develop multiple primary cancers than the general population. In addition, studying LFS patients also allows for additional factors such as mutation status to be considered when evaluating cancer risk. Due to the disease’s heritability, family members of afflicted patients often undergo genetic screening, as well, which can allow cancer risk to be estimated based on family members’ characteristics along with individual data. Therefore, in addition to predicting cancer risk among survivors, Dr. Wang’s lab is also investigating the use of LFS data for risk prediction of first and second primary tumors in LFS families [LFSPRO]. Obtaining a better understanding of cancer risk among cancer survivors and LFS patients can allow physicians and genetic counselors to make more personalized and data driven decisions about patients’ treatment and screening plans, enabling them to achieve early detection of first or second primary cancers. [Personalized Risk Prediction]
PI: Wenyi Wang
Department of Bioinformatics and Computational Biology
Wenyi Wang (王文漪), Professor, Department of Bioinformatics and Computational Biology, Division of Basic Science Research, The University of Texas MD Anderson, Cancer Center, Houston, Texas
Curriculum Vitae